Talk: ML Reproducibility: Sources of Algorithmic, Implementation & Observational Variability, 10/29
4-5pm EDT Tuesday, October 29, 2024, online
ML Reproducibility: Sources of Algorithmic, Implementation, and Observational Variability
Kevin Coakley, UC San Diego
4-5pm EDT Tue., 29 Oct. 2024, online
Reproducibility is fundamental to scientific research, as it underpins trust, progress, and credibility. In machine learning (ML), achieving reproducibility is difficult due to variability in algorithms, implementations, and observational factors. This presentation explores key contributors to irreproducibility in ML, including algorithmic factors like hyperparameter tuning and random weight initialization, implementation differences in software and hardware, and observational factors such as dataset bias and data preprocessing. It emphasizes the need to view ML model performance as a distribution, not a single metric or average of results, and clarifies the difference between reproducibility and portability. The goal is to guide researchers on improving ML reproducibility and identifying the critical information necessary for replicating experimental outcomes.
Kevin Coakley is a Computational and Data Science Research Specialist at the San Diego Supercomputer Center and UC San Diego focusing on AI reproducibility. Kevin holds a MAS in Architecture-based Enterprise Systems Engineering and Leadership from UC San Diego and is pursuing a PhD in Computer Science at the Norwegian University of Science and Technology. Kevin specializes in training and evaluating machine learning models for accuracy and reproducibility in applications like image recognition, time series prediction, and natural language processing.
Sponsored by iHARP, the NSF HDR Institute for Harnessing Data & Model Revolution in the Polar Regions
UMBC Center for AI
Tags:
Posted: October 23, 2024, 9:40 AM
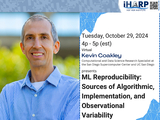