Talk: Rigorous measurement in text-to-image systems, 4/29
4-5pm ET Monday, April 29 in ENGR 231 and Webex
Rigorous measurement in text-to-image systems (and AI more broadly?)
University of California, Santa Barbara
April 29, 2024 4:00 – 5:15 PM ET
ENGR 231 and Webex
As large pretrained models underlying generative AI systems have grown larger, inscrutable, and widely-deployed, interest in understanding their nature as emergent rather than engineered systems has grown. I believe to move this "ersatz natural science" of AI forward, we need to focus on building rigorous observational tools for these systems, which can characterize capabilities unambiguously. At their best, benchmarks and metrics could meet this need, but at present they are often treated as mere leaderboards to chase and only very indirectly measure capabilities of interest. This talk covers three works on this topic: first, a work laying out the high-level case for building a subfield of "model metrology" which focuses on building better benchmarks and metrics. Then, it covers two works on metrology in the generative image domain: first, a work which assesses multilingual conceptual knowledge in text-to-image (T2I) systems, and second, a meta-benchmark that demonstrates how many T2I prompt faithfulness benchmarks actually fail to capture the compositionality characteristics of T2I systems which they purport to measure. This line of inquiry is intended to help move benchmarking toward the ideal of rigorous tools of scientific observation.
Michael Saxon is a PhD candidate and NSF Fellow in the NLP Group at the University of California, Santa Barbara. His research sits on the intersection of generative model benchmarking, multimodality, and AI ethics. He’s particularly interested in making meaningful evaluations of hard-to-measure new capabilities in these artifacts. Michael earned his BS in Electrical Engineering and MS in Computer Engineering at Arizona State University, advised by Visar Berish and Sethuraman Panchanathan in 2018 and 2020 respectively.
Tags:
Posted: April 27, 2024, 9:34 AM
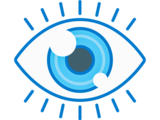